.jpeg)
Navigating MLOps in Traditional Banking: A Journey from Legacy to Cloud-Native ML
In the financial sector, particularly in banking, the transition to modern machine learning operations isn't just a technical challenge—it's a cultural and organizational transformation. As traditional institutions embrace cloud technologies and machine learning capabilities, they face unique challenges that require careful consideration and strategic planning.
The Cultural Shift: From Econometrics to Machine Learning
One of the most interesting dynamics in traditional banking institutions is the existence of sophisticated statistical modeling that predates modern machine learning terminology. Many financial institutions have been using complex mathematical models for years, but they often don't classify these as "machine learning" solutions. This distinction isn't merely semantic—it reflects deeper organizational and cultural considerations.
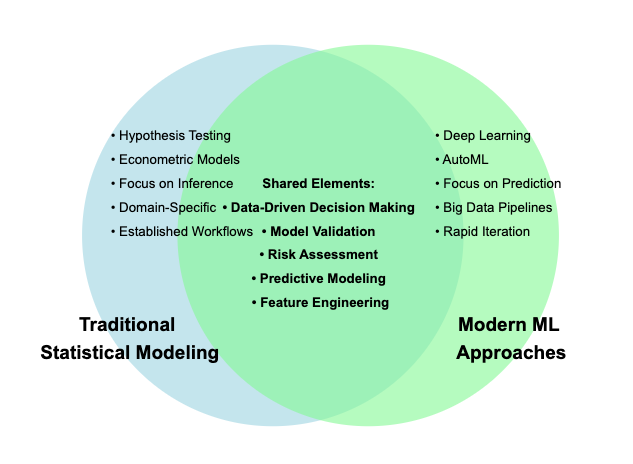
Key observations from the field:
- Traditional statistical models often serve similar purposes to modern ML approaches
- There's often resistance to relabeling existing practices as "machine learning"
- The transition requires careful messaging and stakeholder management
Cloud Migration: The Foundation for Modern MLOps
The journey to modern MLOps often begins with cloud adoption. For regulated industries, this represents a significant shift that requires:
- Careful security considerations and compliance checks
- Gradual migration strategies spanning multiple years
- Balanced approach between maintaining existing operations and innovation
- Clear governance structures for technology adoption
The challenge isn't just technical—it's about managing a controlled transformation while maintaining the institution's core operations and regulatory compliance.
Building an ML Platform Strategy
When building an ML platform strategy in banking, several critical considerations emerge:
1. Abstraction Layers
- Separate infrastructure decisions from ML workflows
- Create clear interfaces between different platform components
- Enable flexibility for future technology changes
2. Vendor Lock-in Considerations
- Evaluate the trade-offs between integrated solutions and flexibility
- Plan for potential future migrations
- Consider multi-cloud strategies where appropriate
3. User Experience
- Account for varying technical expertise levels
- Provide appropriate tools for different user groups
- Balance standardization with flexibility
Compliance and Governance: Preparing for the Future
The European AI Act and similar regulations are reshaping how financial institutions approach ML operations. Key requirements include:
- Complete model lineage and traceability
- Audit capabilities for model training and deployment
- Clear documentation of data usage and model decisions
- Robust governance frameworks
[Suggested diagram: Simple flowchart showing key compliance requirements in ML lifecycle]
Looking Ahead: Building for Scale
For organizations beginning their MLOps journey, the key is to:
- Start with foundational infrastructure decisions
- Build flexible abstractions early
- Consider future compliance requirements
- Plan for gradual capability expansion
- Maintain balance between standardization and innovation
The success of MLOps initiatives in banking doesn't just depend on choosing the right tools—it requires careful consideration of organizational culture, existing processes, and future scalability needs.
Conclusion
The transition to modern MLOps in banking is a complex journey that requires careful planning and execution. While the technical challenges are significant, the organizational and cultural aspects often prove to be equally important. Success lies in finding the right balance between innovation and stability, between standardization and flexibility, and between immediate needs and future scalability.
The key is to build foundations that can evolve with your organization's needs while maintaining the rigorous standards required in financial services. This means thinking beyond immediate tool selection to consider broader architectural patterns and governance frameworks that will support your institution's long-term ML strategy.