Effortlessly orchestrate your ZenML pipelines on HyperAI's cloud compute platform
Streamline your machine learning operations by deploying ZenML pipelines on HyperAI instances. This integration enables you to leverage HyperAI's cutting-edge cloud infrastructure for seamless and efficient pipeline execution, making AI accessible to everyone.
Features with ZenML
- Seamless deployment of ZenML pipelines on HyperAI instances
- Effortless setup and configuration through HyperAI Service Connector
- Support for scheduled pipelines using cron expressions or specified start times
- Smooth integration with ZenML's container registry and image builder components
- Ability to leverage GPU acceleration for enhanced performance
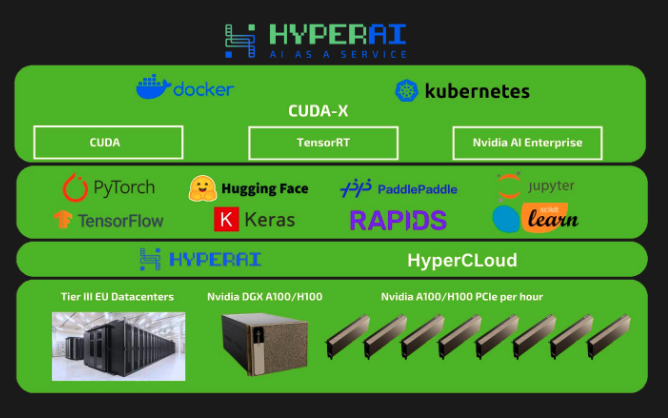
Main Features
- Cutting-edge cloud compute platform designed for AI accessibility
- Docker-based infrastructure for flexible and portable pipeline execution
- Support for GPU-accelerated workloads using NVIDIA drivers and toolkit
- SSH key-based access for secure connection to HyperAI instances
- Managed solution for running ML pipelines without infrastructure overhead
How to use ZenML with
HyperAI
# Register the HyperAI service connector
# zenml service-connector register hyperai_connector --type=hyperai --auth-method=rsa-key --base64_ssh_key= --hostnames=,.., --username=
# Register the HyperAI orchestrator
# zenml orchestrator register hyperai_orch --flavor=hyperai
# Register and activate a stack with the HyperAI orchestrator
# zenml stack register hyperai_stack -o hyperai_orch ... --set
from datasets import Dataset
import torch
from zenml import pipeline, step
from zenml.integrations.hyperai.flavors.hyperai_orchestrator_flavor import HyperAIOrchestratorSettings
hyperai_orchestrator_settings = HyperAIOrchestratorSettings(
mounts_from_to={
"/home/user/data": "/data",
"/mnt/shared_storage": "/shared",
"/tmp/logs": "/app/logs"
}
)
@step
def load_data() -> Dataset:
# load some data
@step(settings={"orchestrator.hyperai": hyperai_orchestrator_settings})
def train(data: Dataset) -> torch.nn.Module:
print("Running on HyperAI instance!")
@pipeline(enable_cache=False)
def ml_training():
data = load_data()
train(data)
# ... do more things
# Run the pipeline on HyperAI
ml_training()
This code snippet demonstrates the setup and usage of a HyperAI service connector and orchestrator within the ZenML framework. It includes registering a HyperAI service connector, creating a HyperAI orchestrator, and setting up a stack. The code then defines a machine learning pipeline with two steps: load_data and train. The train step is configured with specific HyperAI orchestrator settings, including mount points. Finally, the pipeline is defined and executed, allowing the training step to run on a HyperAI instance.
Additional Resources
ZenML HyperAI Orchestrator Documentation
Training with GPUs in ZenML
HyperAI Official Website